The Seventh Conference on Machine Translation (WMT22) is the largest conference on Machine Translation. This year the Unbabel Research team, in collaboration with Instituto Superior Técnico, participated in three different tasks: Quality Estimation (QE), Metrics, and Chat Translation. Here we briefly describe our participation in each shared task.
Highlights:
Our QE system, CometKiwi, was the winning submission of the WMT QE shared task.
Our metrics system, COMET22, was the winning submission for the Chinese-English language pair and the second best for the other two language pairs in the WMT Metrics shared task.
The combination of the kNN-MT technique for MT with fine-tuning, using publicly available data and the development set, led to promising results in the Chat shared task.
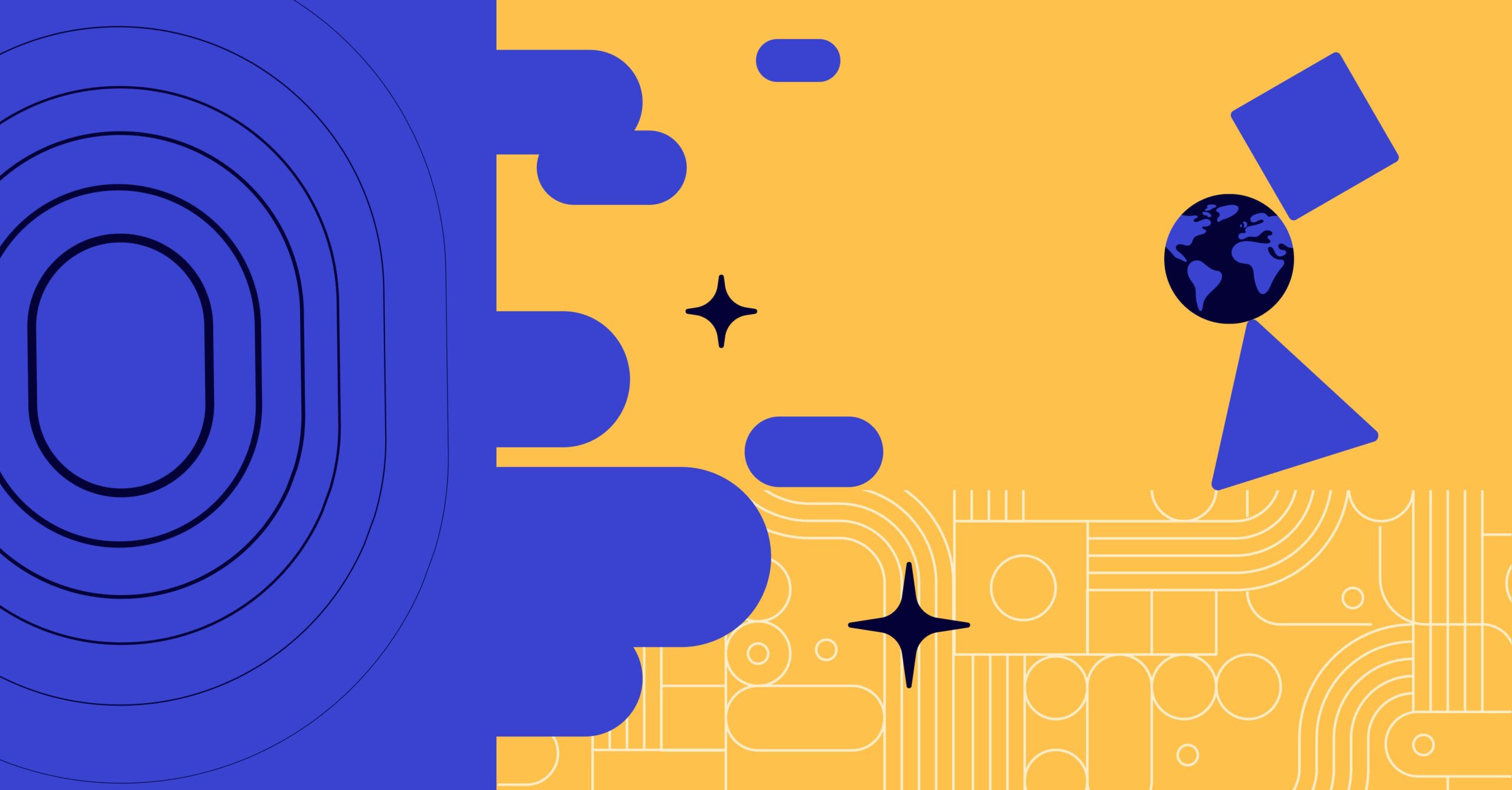
Unbabel and Instituto Superior Técnico researchers at EMNLP 2022 — Abu Dhabi
Quality Estimation
Quality estimation (QE) for machine translation is the process of automatically assessing the quality of a machine translation output without access to a reference translation. At Unbabel, QE is a key component to check which translations are good and which ones need improvement and to route the translation accordingly in order to provide our clients with the best possible translation quality.
Given the importance of QE for Unbabel, we participate in the WMT QE shared task every year, where we compete against other companies and research institutes for the best QE system. Over the years our QE system has ranked first several times and we’re glad to see our updates and improvements keep it as a first-rate system.
The WMT22 QE shared task was split into three different subtasks:
Quality prediction — where systems are tested in their ability to provide reliable sentence-level and word-level quality predictions.
Explainability — where systems are tested in their ability to provide support for their predictions (note that in this subtask participants are not allowed to use any word-level supervision in their models).
Critical Error Detection — translations with critical errors are defined as translations that deviate in meaning, as compared to the source sentence, in such a way that they are misleading and may carry health, safety, legal, reputation, religious, or financial implications. For this subtask, systems are tested in their ability to detect such errors. Check our QUARTZ project to learn more about how we are preventing such errors using QE.
The secret sauce behind our participation this year was the combination of two open-source frameworks that we have been developing over the years: OpenKiwi and COMET. In our submission, we adopted COMET training features — useful for multilingual generalization — along with the predictor-estimator architecture of OpenKiwi to obtain sentence-level and word-level predictions. This combination, which we call CometKiwi, was the best performance system in both subtasks 1 and 3.
For subtask 2, we proposed a new interpretability method that uses attention and gradient information along with a mechanism that refines the relevance of individual attention heads. This method was ranked first for 7 out of 9 language pairs tested, and second for the remaining two languages.
Read the official results and the conference paper describing Unbabel’s submission.
Metrics
Similar to QE, reference-based evaluation is a crucial component of Unbabel’s pipeline. Through Unbabel’s pipeline, the models serving our clients are periodically retrained with more up-to-date content and then tested in several held-out test sets to ensure quality. During that testing phase, the translation outputs from the retrained models are compared with reference translations using machine translation metrics such as COMET. If the new model achieves superior performance than previous versions, that retrained model is deployed.
Given this process’ importance, we participate in the WMT Metrics shared task every year. In this competition, participants compete to be rated the best automatic metric for evaluation with the possible use of references. Happily, Unbabel continued its top-performing streak at this year’s conference.
Unbabel’s submission to this shared task — dubbed COMET22 — is an ensemble between a COMET Estimator model and a multitask model. The COMET Estimator model is trained with Direct Assessments.
The newly proposed multitask model is trained to predict sentence-level scores alongside OK/BAD word-level tags derived from Multidimensional Quality Metrics error annotations. Similar to the QE submission, the multitask model follows a predictor-estimator architecture but takes inspiration from UniTE (a recently proposed metric that was presented at ACL 2022) to work with references.
To ensemble both models, we used Optuna hyper-parameter search to learn the optimal weights between different features extracted from both models. The resulting ensemble was ranked first for Chinese-English and second for the other two evaluated language pairs (English-Russian and English-German) closely following the best-performing metric which was a large-scale model with 6.5B parameters (6x bigger than our ensemble).
Chat Translation
The Chat Translation shared task consists of translating bilingual customer support conversational text. In contrast to content types such as news articles and software manuals, in which the text is carefully authored and well formatted, chat conversations are less planned, more informal, and often present ungrammatical linguistic structures. This year, the task was enriched by the released Unbabel’s MAIA Dataset: A corpus that truly consists of entire, genuine, and original bilingual conversations from four different clients of the Unbabel database, where the agent is speaking English and the customer is speaking non-English The corpus was provided to the participants as development and test sets.
In our role as participants, as we only had access to a development set, the lack of parallel data available was one of the main obstacles to this task. We addressed this by using a large pre-trained multilingual language model, mBART50, which we fine-tuned with the most similar data that we could find on publicly available datasets. Then we performed a second fine-tuning step using the development set.
Following the development of a domain-specific model, we experimented using a data-retrieval approach (kNN-MT) to further incorporate domain-specific data during the decoding process, by searching for similar examples on a datastore (built with the same data that was used to fine-tune the model). The full conference paper describes our submission.
Conclusion
All in all, the team had successful participation in the WMT22 conference and was able to showcase their advancements in Quality Estimation, Metrics, and Chat Translation — winning the WMT QE shared task and the Chinese-English language pair for the WMT Metrics shared task. Not only is the WMT22 conference an excellent opportunity to test our technology against the best in the industry, but it underscores the value Unbabel puts on delivering quality translation to its customers, helping them break down language barriers with their global audiences.