One of our most important jobs here at Unbabel is to deliver high-quality translations. But how do we know if a particular translation is high quality? Different people may have different views of what constitutes a good translation. Even the same person can have a different perception of the same translation, if asked to evaluate it a few weeks apart. Many factors contribute to the subjective nature of translation: people’s language is shaped by where they grew up, the language of their parents, the books they read… and simply by everyday communication.
As a professional translator myself, when working as a reviewer on big translation projects, sometimes it was not easy to make the distinction between what was wrong from what was just written in a way that was not the way I would have written it. In fact, what feels idiomatic to me may not feel idiomatic to someone else, even when we hail from the same country — they might represent a different region, generation, or social class.
I found that having instructions, with concrete examples, of the kind of changes that were unnecessary when reviewing helped me better understand the scope of my work. So did being fully aware of the project specifications, particularly understanding who the audience of the translation was, so I didn’t spend time making changes that objectively did not improve the quality of the translation.
This inherent subjectivity —after all, there is no single right translation— brings about big challenges when the goal is to improve the quality of the translations produced by our machine translation systems and perfected by our community of editors.
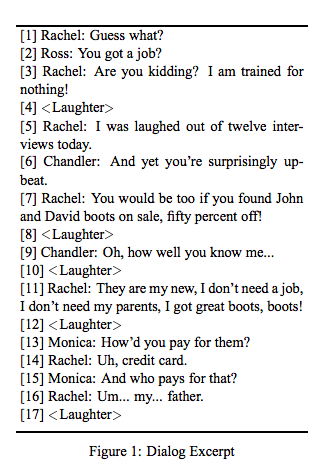
Multidimensional quality metrics: a framework
Surely identifying a bad translation is easy enough, right? We’ve all laughed at machine translation blunders, such as when Google Translate mistook “Ooga Booga Wooga” for Somali, or when a Hotel in the capital of Iraq’s Kurdistan tried to translate the meatball option at a buffet — which, having no direct equivalent in Arabic, was transliterated as ميت بول, and accompanied by this alarming English translation: “Paul is Dead.”
But machine translation technology has improved tremendously in recent years, and it’s becoming harder to find such striking mistakes. Often, they’re a lot more subtle. For example, when you type “Lo pillaron conduciendo a 120 km/h” into the interface of a free, online machine translation system, the translation comes out as “He was caught driving 70 mph.” It looks good! It even converts the units. But 120 km/h is actually more like 75 mph; this is a mistranslation that can seriously impact the quality of the final translation.
It’s not uncommon for state-of-the-art neural machine translation to produce texts that read very well but have a different meaning from that of the original text. But we’re not above reproach — to err is (also) human, and even expert translators sometimes make mistakes.
So, to identify areas of improvement, both in our machine translation systems and in our community of editors, and drive both towards excellence, we need an effective and precise method of assessing translation quality. For us, that’s provided by the Multidimensional Quality Metrics (MQM) framework, developed as part of the EU-funded QTLaunchPad project, aimed at reducing global language barriers.
MQM provides a comprehensive, hierarchical, flexible and standardized system that allows us to pinpoint and address translation quality issues. Specifically, MQM provides an extensive typology of issues, a set of severities, and a scoring mechanism to quantify translation quality.
Based on the specific requirements of a translation project, such as the purpose or the audience of the text, MQM allows us to define a custom quality metric, with more or less granularity. This is useful for those cases where a customer is not interested in certain problems, for example those related to punctuation. When such a case occurs, we can tune MQM to not take those issues into account. MQM allows us to measure what matters to our clients and tailor the notion of quality to theirs.
Our approach
With a quality metric defined, expert linguists conduct error annotation on our own annotation tool. The annotation process involves, for each error encountered, first, highlighting the span of the error; then, classifying it from the list of issues of such a custom metric, and finally, assigning it a severity. At Unbabel, we use an MQM-compliant metric, with the following top level categories, each containing its own set of subcategories:
Accuracy
This dimension characterizes issues that have to do with how well the translation conveys the meaning of the source text. There are some infamous accuracy issues that resulted in confusion… or hilarity. For example, when Steven Seymour, who was U.S. President Carter’s translator in a visit to Poland in 1977, translated his being happy to be there as being “happy to grasp at Poland’s private parts,” as reported by Time magazine. In this case, the only damage was to Carter’s — and the translator’s — reputation, but these mistakes can lead to serious failures in communication, and, some argue, may have even contributed to the breakdown of political relationships in times of war.
Fluency
Fluency is about how natural the text sounds in the target language. Fluency issues can happen on any content, not only translations. These movie titles contain a bunch of Fluency issues, some of which are definitely deliberate.
Style
Style issues occur when the translation doesn’t comply with the specified requirements regarding register or terminology. Getting the politeness level wrong when addressing a Japanese customer is perceived as very offensive. Terminology issues fall in this category too: for instance, using Trash instead of Bin in a MacOs context may result in misunderstandings when providing technical support.
In addition to categorizing issues along the above three categories and its subcategories, our expert linguists assign each issue one of the following three severities: minor, major and critical.
Minor issues don’t have an impact on the purpose or understandability of the content, but they may make it less appealing. For example, in Spanish, the recommended way of translating a percentage like 20% is 20 %, with a space between the digit and the symbol. If a translation doesn’t respect this, it can still be a fit-for-purpose and understandable translation.
Major issues affect the purpose or understandability of the content. An example of a major issue would be a grammatical error that makes a sentence difficult to understand, but where the overall goal of the source text is kept in the translation. Think of a chat conversation where the closing sentence is, “Let me know if there’s anything else I can you with, any time!”
Critical issues differ from major issues because they result in negative outcomes. They render the translation useless, and may carry health, safety, legal or financial implications, or could be seen as offensive. For example: imagine, when providing warranty information to a customer, that the (US) English original says the expiration date is 11. Dezember 2020y (12. November 2020y). If the translation into Spanish says 11. Dezember 2020y (0. Dezember y, 11, 2020), the customer may lose their legal warranty rights because they think they have more time to make a claim than they actually do.
Each of the above severity levels is associated with penalty points, which are then divided by the overall number of words in translation. Then, a simple formula takes into account the count and severity of the issues and the length of the text then gives us a numerical measure of the translation quality based on the specifications set up at the beginning of the project.
It’s getting better all the time
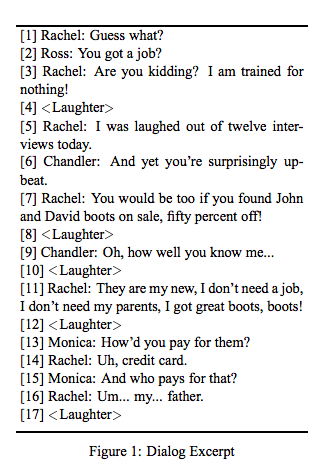
Because MQM is heavily standardized, using an MQM-compliant metric helps mitigate subjectivity in assessing translation quality. But as many in academia and the industry know, no metric makes subjectivity completely go away.
For instance, if we have “I love Lisbon” in the source text, and the translation reads lisbon, without a capital L, what kind of error are we seeing? Is it an entity issue or a capitalization issue?
We’re always working to reduce this inevitable subjectivity, guiding the annotation process by providing linguists with extensive annotation guidelines and training materials with examples. We are constantly in touch with them to help them resolve doubts when they appear or clarify issues, and these interactions, in turn, help us improve our guidelines so that over time they become better and clearer.
Overall, MQM has proved to be a very useful framework to assess translation quality in a systematic way, by letting us identify complex linguistic issues and act on them. But no matter how many formulas and guidelines we develop to control the process, the peculiar ways in which we use language, its subjectivity, its quirks, means translation will always be part science, part art. And we wouldn’t have it any other way.
The post If language is subjective, how can we measure translation quality? appeared first on Unbabel.