AI solutions are no longer the future — they’re here. Microsoft-backed ChatGPT entered 2022 with a bang, harnessing the full power of responsible AI principles and accumulating 1M+ users within the first week of its debut. Despite its impressive new text-generating algorithm, major concerns have arisen surrounding its truthfulness, and how it could lead people to believe in false information — ultimately resulting in real-world harm.
One of the main problems in fully relying on AI systems is that AI doesn’t know when the data is incorrect or even when it shouldn’t be trusted. Predicting an AI algorithm’s quality is one of the pillars of responsible artificial intelligence, but is an area where research is in its infancy, and data scientists are still a ways off from cracking that code.
However, measuring quality is key for building trust in AI technology, and making it a safe and useful environment in people’s lives. The use of AI is incredibly powerful, but it can be unpredictable. It’s like having a genius advisor who knows everything but lies 10% of the time. And the problem with that is you don’t know which 10% of the time you are receiving. But what if you could know, and during that 10% send the information to a data specialist?
During the translation process, quality is paramount and is something that every provider claims to have, but very few are able to demonstrate it in a consistent and systematic way when asked.
For that reason alone, Unbabel has dedicated the past 5 years to developing a sophisticated Language Quality Assessment framework, powered by a community of professional linguists, to ensure we have full visibility of the quality that we are delivering. Our goal is not only to provide full transparency to our customers, but also to collect continuous data to improve our AI systems.
We use it to understand where our AI machine-learning models are falling short, or to identify which freelancers need to improve and in what areas.
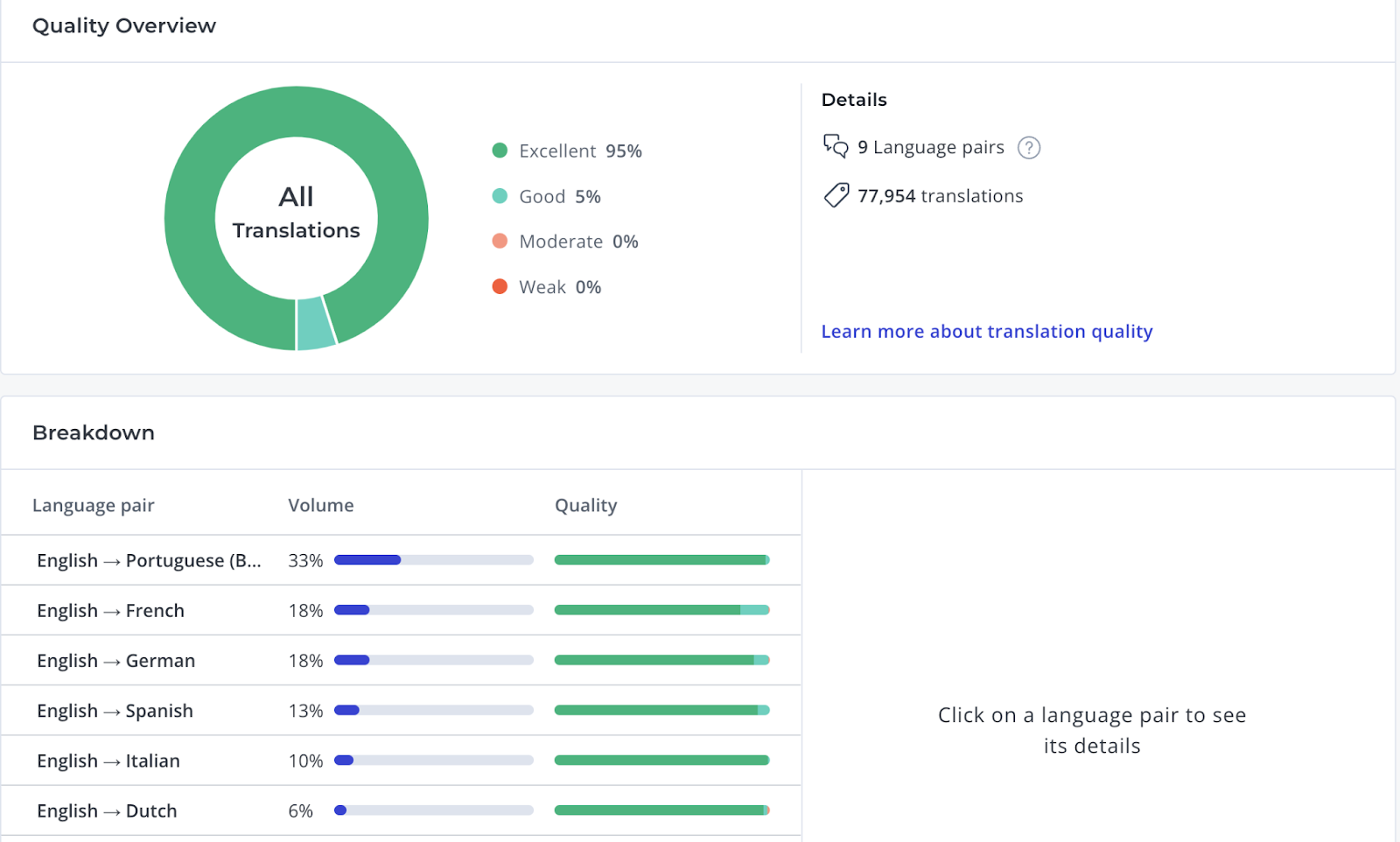
Here’s a look into our MQM annotation product and our Customer Utility Analysis Metric
With that said, Linguistic-made LQA suffers from the drawbacks that translations do: Lack of scalability and high cost even at a greater scale — considering professional linguists that can reliably conduct annotations are a rarer resource than professional translators.
At Unbabel, we understand that to fulfill our vision of building the translation layer, we need to invest in an API solution that provides consistent translation quality at scale. We’ve been employing quality estimation since 2017 to arbitrate when a machine translation achieved sufficient quality for a particular use case, or when it required human intervention for revisions. This allowed us to solve the issue of scale for a particular set of use cases.
In previous iterations, QE quality was not robust enough to be used on human output — there was no intervention to prohibit us from sending a translation to the customer when the quality was not up to par. But recent advances in our human-centered AI technology have allowed us to overcome that shortcoming, estimating exactly how good the quality of the translation is, and enabling us to action it. Our new QE achieves a big correlation with human MQM; we now have the ability to use QE everywhere, which will only open doors to a lot of new use cases.
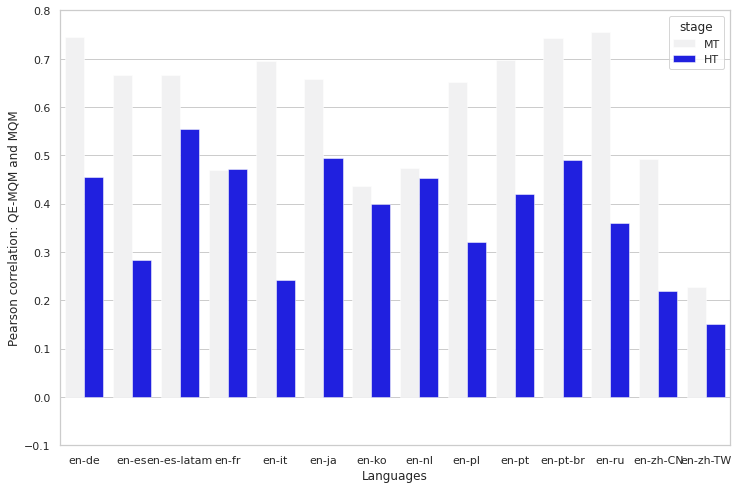
We’re now inviting you to experience the quality for yourself! Try our new QE for free here, and instantly receive a quality assessment of your current translations, among many other features.
Online Quality Assessment
This determines whether we need to refine an existing translation both after MT or after a human translation. Until now, most of the industry relied on the 4-pairs-of-eyes approach of having a reviewer after a translator. This was performed despite the fact that the translator was capable of providing a perfect translation and still did not guarantee quality.
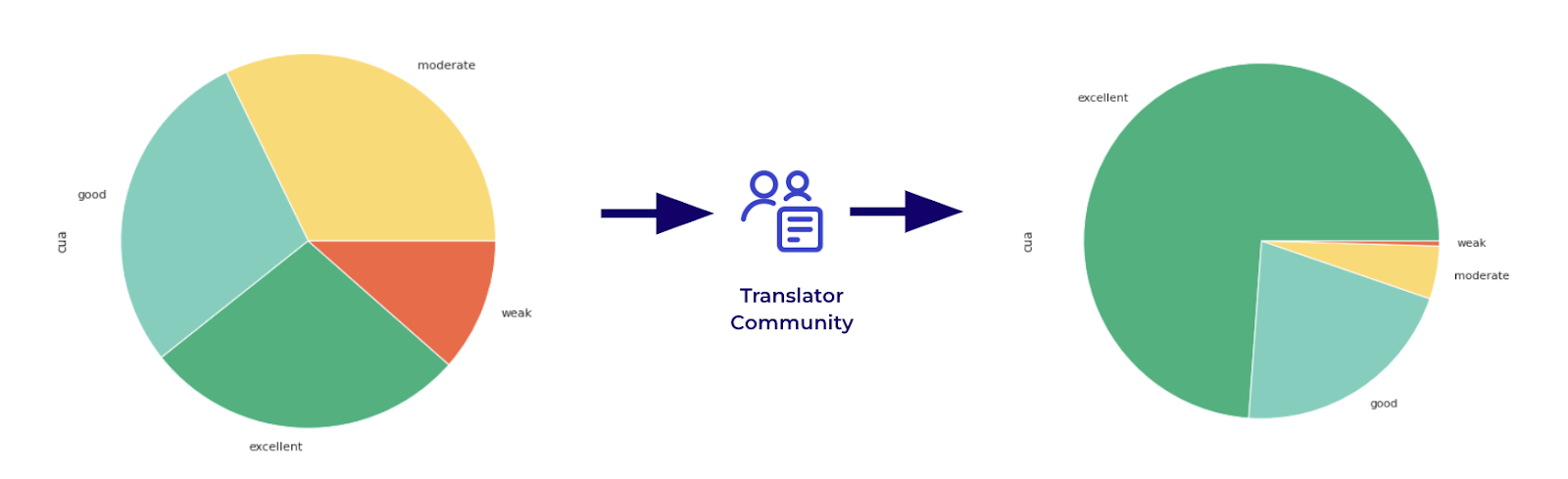
The plot above shows us an example of what jobs were identified and deemed necessary to send to our translator community, but even then the quality wasn’t perfect. The continuous quality assessment allows us to determine whether we want a different pair of eyes for jobs to elevate to excellent status.
Translation Memory Curation
Linguistic errors are bad for business in any translation company; they are even worse when they reach a translation memory and get propagated over and over. Curating large translation memories can be a daunting and expensive translation task, especially when a change in the customer style guides affects the entire collection, such as when the customer’s register preferences change from formal to informal. This doesn’t have to be hard anymore — you can now use QE to scan your entire translation memory, pinpoint what entries are problematic, and get an extra pair of eyes for review.
Machine Translation Data Cleaning & Filtering
Neural machine translation quality is closely tied to the quality of the data that is provided; the old maxim of “the more data, the better” for Machine Translation has consistently proven to be untrue for these models. Instead, the quality of the data is the determining factor for the machine translation quality. This is a general trend in neural machine learning models, and gave rise to a new paradigm of data-centric AI, led by Andre Ng. Until now, filtering big parallel datasets was difficult, and only basic heuristics like sentence length or language identification were used. QE changes the game and allows us to filter training data for our models in a better way. In fact, we observe an average gain in quality of 1.9 COMET points when using QE as data filtering.
Supplier Management
Managing large supplier tools is hard, especially when we add subject matter expertise requirements to the mix. Freelancer managers or other key stakeholders typically rely on onboarding questionnaires but have little information about the ongoing quality of their freelancers. With our QE toolkit, you can receive an ever-updated view of the quality of the freelancer per subject matter, per customer.
Previous Translations Audits
Conduct a quality audit of your past/current translations to see if they are optimal, by running QE over your website, knowledge base, and manuals. By leveraging predictive AI models — built from millions of corrections from past translations and verified by humans — you can rest assured that poor translation quality doesn’t consume your content.
Start your free 14-day trial with Unbabel’s QE, and instantly start setting up your translation pipelines: