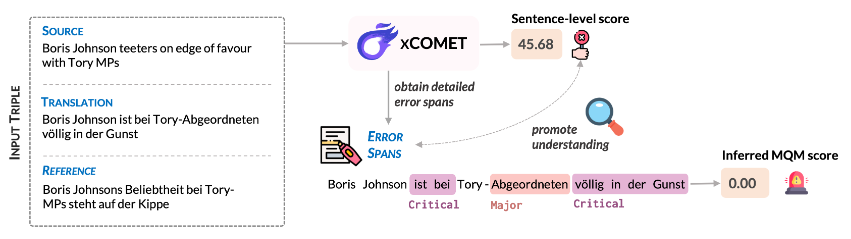
Automatic metrics for machine translation evaluation have come a long way, with neural metrics like COMET (Rei et al. 2020) and BLEURT (Sellam et al, 2020) leading the charge in improving translation quality assessment. These metrics have shown significant advancements, particularly in their ability to correlate with human judgments, surpassing traditional metrics like BLEU (Papineni et al. 2002). However, these metrics, while powerful, have their limitations, as they provide only a single sentence-level score, leaving translation errors hidden beneath the surface.
In an era where large language models (LLMs) have revolutionized natural language processing, researchers have started to employ them for more granular translation error assessment (Fernandes et al. 2023, Kocmi et al. 2023). This involves not just evaluating the translation as a whole but also pinpointing and categorizing specific errors, providing a deeper and more insightful view into translation quality.
Here is where XCOMET makes its grand entrance. XCOMET is a cutting-edge, open-source metric designed to bridge the gap between these two evaluation approaches. It brings together the best of both worlds by combining sentence-level evaluation and error span detection capabilities. The result? State-of-the-art performance across all types of evaluation, from sentence-level to system-level, while also highlighting and categorizing error spans, enriching the quality assessment process.
But what sets XCOMET apart from the rest? Here’s a closer look at what makes it a game-changer:
- Detailed Error Analysis: Unlike traditional metrics that offer just one score, XCOMET digs deeper by identifying and categorizing specific translation errors. This fine-grained approach provides a more comprehensive understanding of the quality of the translation.
- Robust Performance: XCOMET has been rigorously tested and outperforms widely-used neural metrics and generative LLM-based approaches. It sets a new standard for evaluation metrics, demonstrating its superiority in all relevant evaluation vectors.
- Robustness and Reliability: The XCOMET suite of metrics excels at identifying critical errors and hallucinations, making it a reliable choice for evaluating translation quality, even in challenging scenarios.
- Versatility: XCOMET is a unified metric that accommodates all modes of evaluation, whether you have a reference, need quality estimation, or even when a source is not provided. This flexibility sets it apart and makes it an invaluable tool for translation evaluation.
How does XCOMET Compare with Auto-MQM and other Metrics?
Let’s delve into the results to see just how impressive XCOMET truly is. We’ve conducted thorough evaluations, including a comparison with other widely known metrics, including recent LLM-based metrics. Take a look at these two tables:
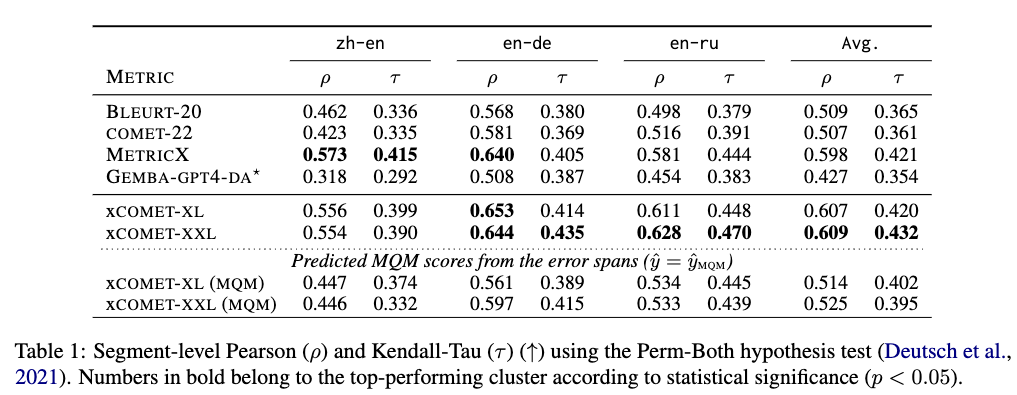
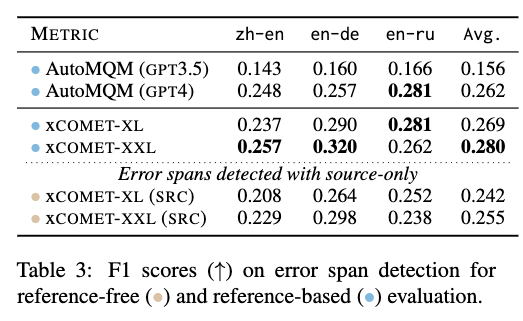
These tables highlight the exceptional performance of XCOMET. In segment-level evaluations, XCOMET outshines other widely known metrics, including the recent LLM-based metrics such as GEMBA-GPT4. When compared to AutoMQM based on GPT-4 at the word level, XCOMET maintains its superiority, even when used without reference in a Quality Estimation scenario!
It’s worth noting that AutoMQM based on GPT-4, while impressive at word level, relies on large and costly LLMs, limiting its accessibility and applicability. XCOMET, on the other hand, outperforms GPT-4 and thrives with cost-effective LLMs like GPT-3, making it a versatile choice for researchers and practitioners in the field.
To make XCOMET accessible to the community, we have released two evaluation models: XCOMET-XL, featuring 3.5 billion parameters, and XCOMET-XXL, with an impressive 10.7 billion parameters. These models are available through the COMET framework and the Hugging Face Model Hub:
Connecting to Unbabel Quality Intelligence
XCOMET shares its foundational technology with UnbabelQI, with a key distinction being UnbabelQI’s utilization of proprietary MQM annotations, spanning an impressive array of up to 30 languages, and its ability to seamlessly adapt to diverse customer domains and expectations. While XCOMET excels across various evaluation scenarios, UnbabelQI is primarily tailored for reference-free quality estimation. In real-world, ‘in-the-wild’ situations, reference translations are less common, making UnbabelQI an ideal choice for assessing translation quality in such scenarios.
In a world where language and translation are more critical than ever, Unbabel is committed to revolutionizing the way we evaluate translation quality, providing a level of insight and performance that was previously unattainable.
To learn more about Unbabel’s QE capabilities and LangOps, visit our platform and our UnbabelQi demo here.