Imagine sitting in a circle with a few people where each of you knows only two languages — one shared with the person on your left, and one shared with the person on your right. If you say something to the person on your right and ask them to pass on the message, it might very well be that, after being passed along all the languages, it comes out sounding very different from the original message.
This might seem like a very weird game of Telephone to you, but in the same way that whispering impairs your ability to hear the message, so translation works as an imperfect communication channel. When you try to translate a message into a different language, you can change its intended meaning without being aware of it. Oftentimes messages are subjective, ambiguous, or, in some cases, even impossible to represent without any loss of information.
But why is translation such a challenge? And in being so, can we ever achieve such a thing as a perfect translation?
The weight of words
Translation is not the same as localization. It’s seen as a more objective task, where you look to obtain the closest meaning possible of a given text, in a different language. Localization, on the other hand, carries the weight of adjusting the message to the target culture.
Let’s assume for a second that this is true. As a translator, you still need to decide on the grammatical structures, lexical choice and linguistic style to use, and this isn’t always a straightforward process. Some languages omit parts of speech that for others are essential to a fluent text. Some agglomerate words, others rely on inflection of terms. Some have grammatical genders, and some do not.
When it comes to lexical choice, there are words that do not translate well into other languages. Many concepts may not have an equivalent in the desired language, and others may be abstract and incredibly difficult to put into words. It’s the case of the Portuguese word saudade — which means more than missing something or someone, longing for them or feeling nostalgia. Or the case of the Danish hygge — which is not just sipping a hot beverage under a cozy blanket while it snows outside (although, if you ask me, that already sounds pretty good!).
This does not happen with the majority of words, but it is nonetheless evidence that we don’t have all the same concepts across all languages. Another difficulty is when several words translate into the same word in a given language. In one direction you might end up with repetitive text, and in the other, you might not know which word to pick. Even the simple word “you” can be a challenge.
Then there is creative text, from its simplest forms to literary compositions. There is a fine line between art and science, and the decision to either keep the text as close to the original or to explore the meaning the author intended to convey is not an easy one. In this process, according to Antoine Berman, translators can incur in a number of tendencies that deform the text. There are twelve of these tendencies.
One of them, to a certain extent inevitable, focuses exactly on the loss of lexical diversity mentioned above. It is called quantitative impoverishment. And among other sins like expanding too much on a subject, or resorting to the translation to clarify details that were not clear in the original, there is also the destruction of other linguistic features, such as the rhythm of the text. One example of this phenomena is one of my favorite monologues.
However, this valorous visitation of a by-gone vexation stands vivified and has vowed to vanquish these venal and virulent vermin van-guarding vice and vouchsafing the violently vicious and voracious violation of volition. The only verdict is vengeance—a vendetta, held as a votive, not in vain, for the value and veracity of such shall one day vindicate the vigilant and the virtuous.
Alan Moore in “V for Vendetta”
Let me try to paint a picture here. If I go about changing a bunch of the words, I can easily destroy the rhythm that makes the text so strong and relates to the character V and his vendetta.
However, this bold visitation of a by-gone annoyance stands revived and has promised to end these corrupt and pernicious pests van-guarding sin and vouchsafing the intensely cruel and avid transgression of free will. The only conclusion is vengeance—a revenge, held as a votive, not in vain, for the value and veracity of such shall one day absolve the wary and the noble.
It should be easy to see how a similar replacement could prevent the rhythm in the original text from surviving in a translation. This and other linguistic phenomena pose a huge challenge. They are not just an instrument of beauty, but can also be essential tools in delivering the intended message.
It goes without saying that we need to choose our words very thoughtfully. Words carry meaning, and when you choose them wisely they can be extremely powerful. However, when you choose them carelessly… Let’s just say it can lead to really bad situations.
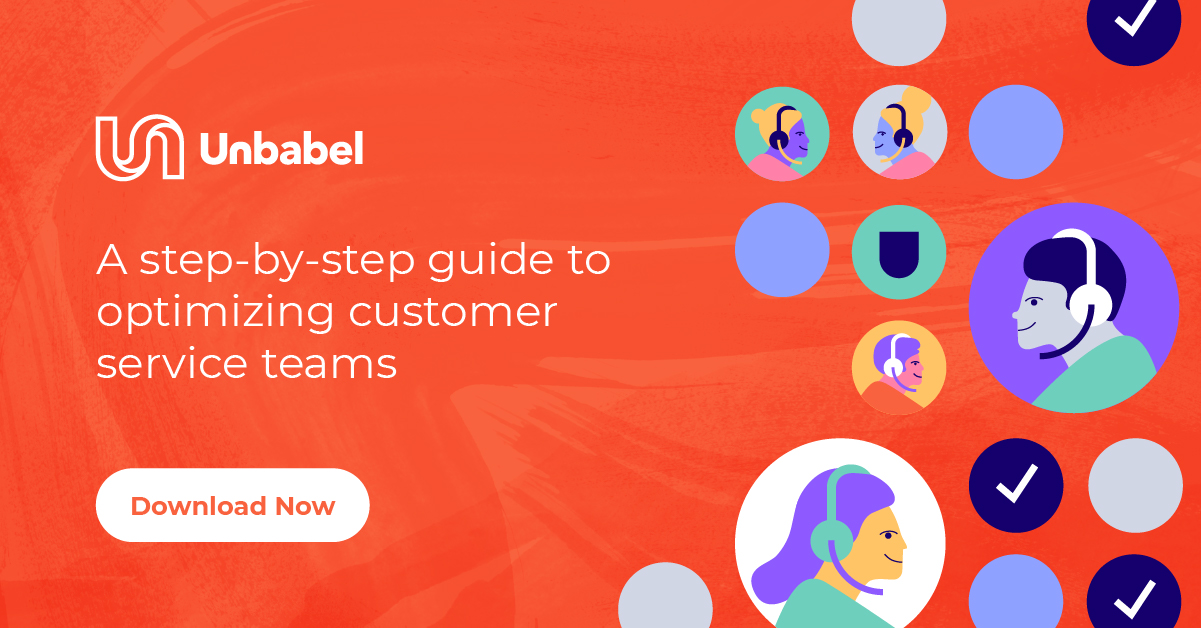
Culturally speaking
I presented translation as a separate concept from localization. But localization plays an important role in translation. One can only translate without adapting to the target audience as a theoretical exercise. In any practical application, we need more.
The most basic forms of localization rely on adjustments related to symbolic representation of entities such as numbers or dates and currency formats. It includes other specific aspects, such as the sorting and positioning of elements, and the more sensitive task of avoiding concepts or ideas that might be misinterpreted or offensive by the target audience. But this is just a small part of what culture means for translation.
We can not really talk about language without diving into culture. The two walk hand in hand. Language shifts with cultural changes and revolutions, but it can also be used as a mechanism to influence and guide culture. Thus, the cultural aspects of a society are evident in their language — in the available words, in the typical expressions and in the constructs used.
Language is the Roadmap of a Culture.
Rita Mae Brown
When learning a new language, you might start with the alphabet and vocabulary, its grammatical structures and linguistic rules. But you’ll often see that most teachers and books will add information on important customs and behaviors of the culture. These often provide an explanation for patterns and rules that would otherwise feel arbitrary.
One such example of this intertwining is social hierarchy and its importance in the different tones and styles. To fully understand how to address someone, you need to not only know the available tones of a language, but also when to resort to each one of them. And even though some cultures share similarities, these rules are not universal.
Take Sweden, for example. If you were to ask a translator to provide two versions of a dialogue with distinct levels of formalities, you might be shocked to see very few or even no differences. But that would nonetheless be correct. Nowadays, the Swedish language is quite informal, and you would not use titles or formal words to address anyone. You just address everyone as “you”. And it is all thanks to the du-reformen (you-reform), a moment that shifted the Swedish culture, reducing the importance of class distinctions and, alongside it, the Swedish language.
Conversely, in Japan, there are several layers of formality and politeness, and navigating through them might be tricky. Japanese culture is deeply rooted in strong values of respect and humility. These transpire into the language, in concepts such as 尊敬語 [sonkeigo] and 謙譲語 [kenjôgo]. Sonkeigo is a way of elevating the person you are talking to, kenjôgo is used to lower yourself. Another example is the word 先生 [sensei], which is used when referring to or addressing a teacher. However, refer to yourself as a sensei and you may come across as conceited or even impolite.
Another aspect in which culture impacts language is by feeding the lexical issues described before. Culture is what causes some concepts to be so well defined, while others are not. Even geography has an impact on this. You don’t need to go further than the north of Europe to see it.
The Sami are an indigenous people from the northernmost regions of Scandinavia. They have over 200 words for snow — right up there with reindeer and ice. And they’re not alone. Swedish, Finnish, Russian, among other languages all have several words for it. It is obvious that the weather is vital to these communities, and it may be a matter of life or death to know that you should avoid sabekguottát — a frozen crust that just about carries your skis, but breaks if you have to do anything else — but that in tjarvva — untouched, hard, really crusty snow — it’s safe to migrate your reindeer.
There are many other ways in which culture mixes with language, and it is not easy to dissect how they influence each other. We don’t know for sure if one is pushing the other or vice-versa. But we do know they evolve somewhat together and that they are connected. And it may even shape the way we think, in ways that we are not even aware of. In his book “1984”, George Orwell toys with this concept by showing how it could be used to control and limit thought. Maybe we can think in different ways and go beyond language, and maybe thought drives language and not the other way around. Either way, it definitely limits how you can share those thoughts with others.
But if thought corrupts language, language can also corrupt thought.
George Orwell
Lost in (machine) translation
In recent years, the efforts to improve machine translation have proven extremely successful, and we’ve seen huge jumps in performance, in particular with the introduction of neural networks. A second wave of improvements is in progress, and as new neural network architectures arise and huge models are released into the wild, we see over and over claims of human parity rise up. But are we really there yet? What are its limitations and how are we tackling them?
The truth is machine translation still has a lot of shortcomings when you look into how it works. First, it does not look into context. Most models nowadays look only into single segments. In an attempt to reassess the claims done, Antonio Toral pointed that the setup itself focused only on assessing sentences individually, ignoring inter-sentence phenomena. This approximates the setup to the scope of the machine and away from real translation scenarios, where the final deliverable is the whole document.
There is, however, some work looking into integrating context in this task, often referred to as document-level machine translation. By looking into specific phenomena like lexical and grammatical cohesion, but also meaning coherence and discourse connectives, new techniques try to integrate context to optimize these factors. So far most work is limited to short spans of context, and it still doesn’t account for longer dependencies, but it has shown that even a small window of context helps, and it continues to evolve with every new technique.
Additionally, even as some claim human parity, others have seen evidence of deformations in machine translation that are similar to the ones a translator should be aware of. One example is the loss of lexical diversity, the same quantitative impoverishment that is a challenge even for translators, but that they, if careful, can avoid. In this work, Eva Vanmassenhove and her colleagues propose that the data-driven aspect of this task leads to a preference over specific translations and the loss of other variants, decreasing the richness of the generated sentences.
This loss might even reveal biases in the data, including ones we are already familiar with. Gender bias is one of such topics ( “He’s a doctor. She’s a nurse”) and there are techniques aiming specifically at tackling it, such as integrating dedicated tags to guide the translation. But these tags are not always available. They might be embedded in context or need to be explicitly provided. And even though we are more aware of these flaws, this is still a pretty unexplored space, and not many real systems are able to fully cope with this loss.
Finally, the data you have available can vastly limit your performance. Machines learn by generalizing over the data they receive. But not all data out there is amazing. This noise can impact the machine translation in unexpected ways and lead to huge errors. Moreover, neural models can overfit to the data, in more than one way. And when it does so, it can be hard to detect, mostly because neural machine translation outputs usually appear fluent.
These misleading outputs are usually caused by the model overweighting some of the most common examples in detriment of others. This is even more common when you try to fine tune to a specific domain. There is a sensitive tradeoff between specialization and generalization when it comes to statistical models, and neural networks appear to still suffer from a lack of robustness when presented with unexpected inputs.
I have focused on the challenges of translation, assuming it is a well described task. But one of the biggest challenges for AI when we talk about language generation is knowing what to optimize for. Translators don’t really agree with each other when it comes to evaluating their peers’ work, making it extremely hard to understand what the final goal is. Ironically, in the task of objectively replicating a message in a different language, each person has their own version of the best way to do so.
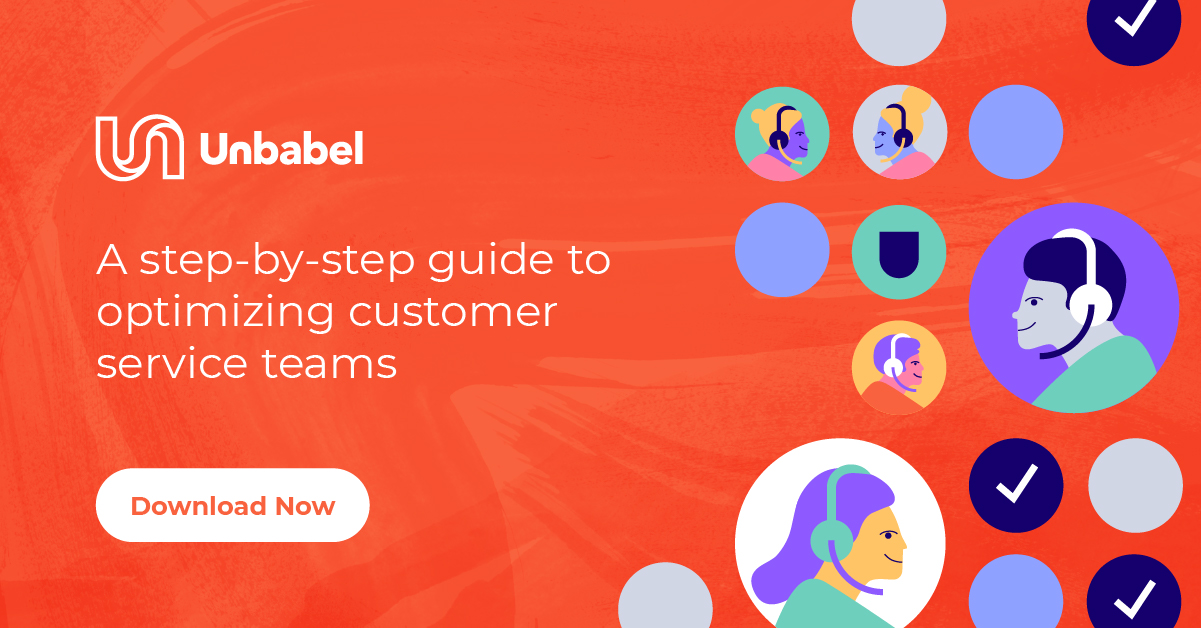
The rise and fall of English
Claims of human parity are constantly refuted and re-affirmed, but if you look closely, you’ll see they are mostly made in scenarios where English is present. Indeed, up until now, the majority of online content was in English, and this has mostly meant translating content both from and into it.
But the internet is changing. Over the last twenty years, the amount of Chinese-speaking users online has grown about 2600%, almost catching up with English-speaking users. Today, as a second language for many, English still remains widespread, and it is often preferred when trying to appeal to the biggest audience possible. But it may not be so tomorrow.
The reality is, people prefer to access content and engage in conversations in their own language. So to truly provide a world without language barriers, we need to cope with the absence of this language. The problem is, the current models dominating translation not only require parallel data, but they require massive amounts of it. Yet there is little to no data for language pairs without English. So what can we do in this case?
The answer lies in piggybacking on existing data and taking advantage of English as a way of connecting other languages. This is the case of pivoting, where two systems are built for different language pairs with one language in common, and translation is then made in a 2-step approach. Another possibility is a zero-shot approach, where a multilingual model is trained with several language pairs and then is expected to perform in any combinations of the languages involved.
Each comes with its set of challenges.
While having two steps means having two points of failure, and has high sensitivity to error propagation, multilingual models, with their internal representations of mixed languages, might still be more unstable, and we often see them produce degenerated outputs. But in the same way neural networks stood to their more understandable counterparts — phrase based systems — so the rise of multilingual systems may be closer than we think.
For both, however, with very little to no data available to validate and stabilize these systems, any mismatch in meaning or perturbation on the data turns out to be much more serious. Models are coupled with the data available — what you give is what you get. In this scenario, it is even more important to find translators that can help produce better data and validate or even correct these models.
Human powered AI
With all the challenges it faces, one has to wonder — is machine translation ready to stand alone? Or are we still far from releasing it into the wild? The answer may lie somewhere in between.
Despite the efforts to ease the several shortcomings of automatic systems, we can not claim that translation, as a whole, is solved. Whether you can use machine translation exclusively or not, it all comes down to your use-case. For example, use-cases with common content and a lot of repetitiveness can be easier to tackle, while technical or literary texts are still far from being fully solved. And in the end, this is exactly what we want — to get the repetitive out of the way and the boring automated.
In other applications, it has even proven to help translators be faster. The resulting sentences, generated by machine but edited by translators, are called post editions. However, some have argued that they suffer from some of the same afflictions that are seen in machine translation. Translators need to be increasingly aware of this, so that they don’t get biased and miss the deformations of the underlying systems.
But will we ever get to a point where humans are obsolete?
Some claim that AI is making language learning obsolete, but I would say it still remains very much the opposite. While in some cases you can probably get your machine to translate deceptively well, it still cannot do it without data. Machine translation is still very much a supervised task, which means we rely on actual human-generated data to train these models. As Andy Way put it, “the translator will always be the human in the loop.” This will continue to be true in this paradigm, both as the teacher and as the judge of the machine.
There is still a lot to translate, and a whole world of language to tackle. Our vision at Unbabel is a world without language barriers, and we believe that in order to make it happen, we need to bring humans and machines together. And even if it is not possible to be fully accurate in translation, with proper care and consideration, it can definitely help to connect different people and build understanding. And maybe help us be better at multilingual Telephone.
The post A game of Telephone: how accurate can translation really be? appeared first on Unbabel.