Unbabel releases the first large language model (LLM) specialized in predicting the quality of a translation to the public, the first of a series of LLMs that the company is currently working on.
During the last few years, machine translation has come a long way, with performance that has sometimes been thought of as achieving human parity (Hassan et al. 2018, Popel et al. 2020). However, several works have analyzed these claims, considering more challenging domains, expert evaluation, and context, and have found that machine translation still lags behind humans (Laubli et al. 2018, Freitag et al. 2021). This means that we still can’t fully trust AI to automate translation in a business environment. There are also so many machine translation models on offer that have varying levels of performance depending on domain and language pair, it’s daunting for businesses to ensure quality meets requirements.
This is where Quality Estimation (QE) comes to the rescue. Quality Estimation is the task of predicting the quality of a translation without access to a reference translation (Specia et al. 2018). In today’s world, this is achieved by training specialized LLMs to detect when the machine translation system fails to produce the expected quality. This can then be used to request human intervention when necessary, helping recover from machine translation errors and making the entire machine translation process more efficient and reliable. This is crucial for deploying AI at scale in a safe manner and building trust among users.
Today, we are excited to introduce CometKiwi XL (3.5B) and CometKiwi XXL (10.7B), the open-sourced versions of our state-of-the-art QE model and the first of a series of LLMs that the company is working on.
Named in homage to its predecessor (OpenKiwi), CometKiwi (pronounced Comet-qe) builds upon the foundations established by COMET, showcasing exceptional performance and achieving remarkable correlations with quality assessments. Supporting up to 100 languages, these represent the largest LLM for QE ever released and secured the first-place position in the WMT 2023 QE shared task. This achievement encompassed high-resource language pairs such as Chinese-English and English-German, as well as low-resource language pairs like Hebrew-English, English-Tamil, and English-Telugu, among others.
Why did Unbabel open source our QE LLM?
A key ingredient for AI trust is transparency and by making these models available, our goal is to promote collaboration, facilitate knowledge sharing, and to drive further advancements in quality estimation and machine translation, especially in areas such as reinforcement learning, where a robust QE model is necessary to provide feedback and steer generative LLMs toward high-quality translations.
Unbabel has a long history of open-sourcing its AI models, starting in 2019 with OpenKiwi, its open-source framework for quality estimation, and more recently, since 2020, with COMET, its framework for machine translation evaluation and quality estimation. Our open-source approach offers several advantages, including faster iteration, more flexible software development processes, robust community-driven support and development, and, most importantly, it ensures that when releasing these models, they are tested by researchers all over the world, who contribute with various improvements that we can incorporate and adapt. For instance, after the first release of COMET, researchers from the NLP2CT Lab at the University of Macau and Alibaba Group developed UniTE. UniTE was built on top of the COMET codebase, outperforming the original COMET models and demonstrating greater resilience to issues identified by a group of researchers from the University of Zurich. This group found that the original COMET models struggled to recognize errors in numbers and named entities (Amrhein et al. 2022). These reported problems inspired us not only to improve our current models but also to develop safety mechanisms and test suites for business-critical errors and hallucinations that we now use to test all our models.
Similar to its predecessor from last year, these models are optimized to predict a score between 0 and 1, where 1 represents a perfect translation, and 0 represents a translation that bears no resemblance to its source (e.g., a detached hallucination).
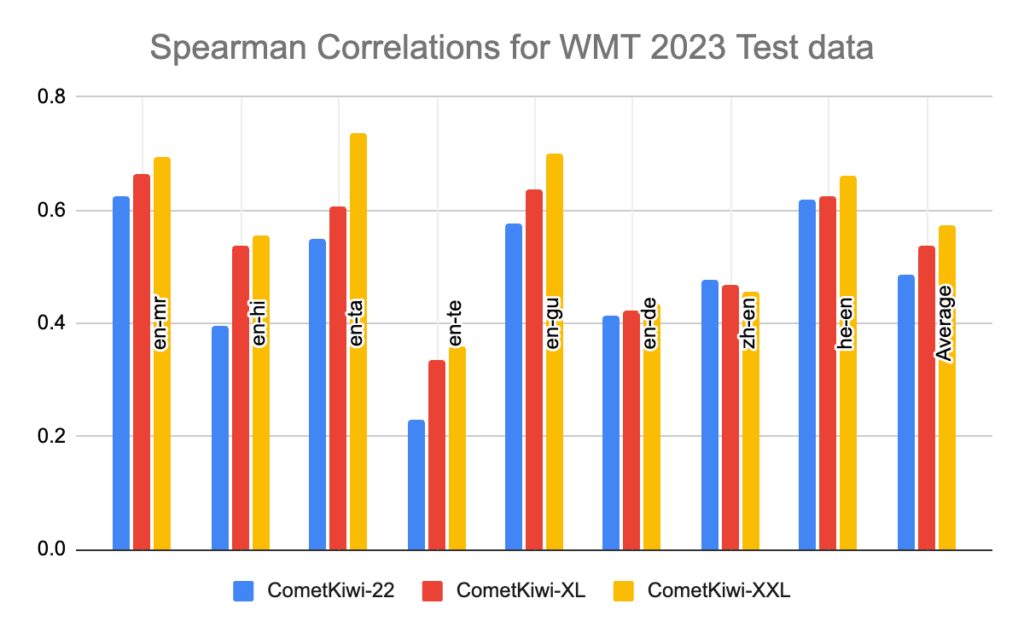
As observed in the plots above, compared to previous versions, CometKiwi XL and XXL achieve significant improvements in terms of Spearman correlations with annotations performed by professionals. These results are taken from our submission to the WMT 2023 QE shared task, the most prestigious competition for Quality Estimation which Unbabel won for the last two years.
These models are available through the COMET framework and the Hugging Face Model Hub:
What’s next? Unbabel will keep working on developing its open-source LLM, and the next release will consist of a larger model that will be state-of-the-art for other multilingual tasks such as translation, NER, and many others.
You can read more about Unbabel’s LLM in our press release here.