At Unbabel, the integration of Artificial Intelligence (AI) with human post-edition (PE) to achieve high-quality translations is our fundamental priority. To ensure that we consistently deliver on our promise, we’ve invested heavily over the years in building out a robust set of technologies that include:
- Machine Translation (MT) engines
- Real-time Quality Estimation (QE) system evaluation
- A visual representation of our quality framework (Customer Utility Analysis)
- In-house Language Operations Platform with its own self-service option
With that, we’ve recognized that the quality of the source text plays a critical role throughout the translation process. Regardless of the robustness of MT systems, the quality of the source text has a major impact on the quality of the translations, mainly when the pipeline has an MT-only flow with no human revision on the final quality of the translation.
This article delves deep into our efforts to enhance translation quality by focusing on the significance of the source text, as well as introducing our customized evaluation framework.
Creating a Tailored Evaluation Framework
In 2020, we secured a major investment in translation technology to understand how the quality of the source text has an impact on the MT. The initial approach was to create an evaluation framework focused only on the source text. While existing translation metrics like the Multidimensional Quality Framework (Lommel et al., 2014), also known as MQM, are widely used in the translation industry, they do not solely target the quality of the source text. Therefore, we created our own evaluation methodology, which enables us to identify patterns in the source text and understand their impact on the translation.
Customer Support Use Case: Agent and User-Generated Content
One particular area of interest for us that presented a unique set of challenges is the translation of Customer Support interactions. We examined both sides of these interactions: the Agent and User-generated content. While agents were typically instructed to follow templated responses and often pigeonholed into communicating in English, whether or not it be their native language, users are unconstrained regarding structure and manner of writing. Through our research (Gonçalves, 2021), we discovered that errors in the source text and linguistic structures commonly found in online languages, such as emoticons, abbreviations, idiomatic expressions, conversational markers, profanities, and message segmentation, significantly impact the translation quality. Building on this experience and additional resources, we developed a production-ready typology called Source Typology (Gonçalves et al., 2022), which classifies and addresses these issues.
Monolingual Use Case: Expanding Beyond Customer Support
Unbabel’s expansion into different use cases, including Localization, posed new challenges due to diverse content types, varying pipeline strategies, and the need to adapt to different target audiences. To address these challenges, we explored the use case of Monolingual Proofreading. Its scope is having a professional translation of any type of content where there is no access to the source text, and verifying if the translation delivered is comprehensible and accurate, while also meeting the standards of its target language and readability expectations of its target audience. Consequently, we adapted our evaluation framework to analyze the quality of monolingual texts without any direct relation to a source text. This expanded typology is now known as Monolingual Typology.
Impact on Translation Quality
At Unbabel, we have made our Quality Intelligence Report (Unbabel Qi) available to everyone, providing real-time translation scores generated by our in-house QE system. To exemplify the impact of source text quality on translation quality, let’s consider an example with a source text containing Grammar, Spelling and Omission errors:
“I would come to Lisbonn in Ø, but Ø haven’t planted anything.”
The following sentence is a Brazilian Portuguese translation from an online MT system:
“Eu viria para Lisbonn em Ø, mas não planed nada.”
Source Text (English) | Error Type in Source | Translation (Brazilian Portuguese) | Error Type in Target | Explanation |
would | Grammar | viria | Grammar | Wrong verb tense |
Lisbonn | Spelling | Lisbonn | Wrong Named Entity | The Named Entity is not translated |
in Ø | Omission | em Ø | Omission | Omitted Noun |
planted | Spelling | planed | Untranslated | The word was left in English |
The table above demonstrates how the majority of errors from the source text were transferred to the translated text or became more severe. This phenomenon is commonly observed in commercially available MT systems, as mentioned in Gonçalves (2021). To further illustrate this point, the image below showcases the translation scores and quality assessed using Unbabel Qi:

The accurate identification of all errors in the translation is evident from the results provided by Unbabel Qi. In addition to error identification, this service uses MQM scores to classify the translation’s quality. Furthermore, Unbabel’s in-house quality framework, known as Customer Utility Analysis (CUA), visually represents translation quality through a 5-bucket color schema, which is a visual representation that helps during the quality assurance process. In this case, the translation is categorized as Weak with a score of 9 MQM, as indicated by the red color.
With all the essential components in place, such as the ability to capture and categorize errors and linguistic structures in source texts, the adaptation and expansion of our evaluation methodology to encompass various use cases beyond Customer Support, and the instant scoring of translation quality, a pivotal question arises: How can we further enhance translation quality?
Automatic Source Annotation and Correction
To continuously enhance translation quality, Unbabel has developed an innovative strategy involving automatic source annotation and correction. This process entails annotating the source text automatically according to our methodology and subsequently applying automatic corrections to the identified errors. By utilizing these corrected source texts, we have observed a significant increase in translation quality. This iterative cycle of merging manual and automatic metrics enables us to make the evaluation process faster while maintaining and assuring the highest standards of translation quality for our customers.
To effectively demonstrate this improvement, the most compelling approach is to provide the previous example with the source text corrected. The translation of the corrected source will originate from the same commercially available MT system. Upon making the necessary corrections, a notable enhancement in translation quality becomes evident:

The sentence above has undergone a complete transformation with zero errors, leading to an increase in the quality score to a 100 MQM score. This advancement signifies a substantial gain of 91 points compared to the previous score. Moreover, this score propels the translation from the initial Weak category to the Best bucket of the CUA framework.
In one of our recent experiments, we observed a significant improvement by incorporating source correction into the translation process. This experiment encompassed 6 language pairs (LPs), all with English as the original language, and used a random sample of customer support data. Each LP involved the evaluation of two datasets: one without source correction and the other with source correction. Subsequently, both datasets were translated with a commercially available MT system.
The resulting translations were then subject to an automatic evaluation using Unbabel’s QE system, which was fine-tuned with in-house MQM annotation data (MQM-QE). This advanced system was developed to predict MQM scores with exceptional precision. Finally, we conducted a comprehensive analysis of the MQM-QE scores across the two datasets, yielding the following final results:
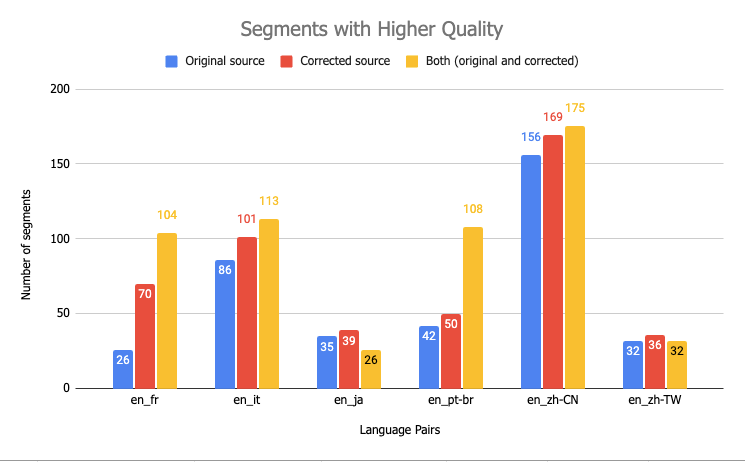
In the figure above, 3 components were taken into account:
- Original source → MQM-QE scores of the translations from the original source data;
- Corrected source → MQM-QE scores of the translations from the corrected source data;
- Both → instances where both datasets had the same MQM-QE scores.
The values displayed in the above figure represent the number of segments, and upon examination, it becomes evident that in all LPs, translations with source correction yielded higher values compared to those without source correction. Additionally, it was common for both datasets to have identical MQM-QE scores. It is important to note that since the data used in this experiment was a random sample, certain segments were initially error-free and thus did not require any corrections. As a result, these segments shared the same scores across both datasets. By combining the two components ‘Corrected source’ and ‘Both’, we can confidently assure the translation quality of our pipeline.
LP | MQM-QE score (average) Original source | MQM-QE score (average) Corrected source |
en_fr | 82 | 85 |
en_it | 88.5 | 90.5 |
en_ja | 83 | 84 |
en_pt-br | 89 | 90 |
en_zh-CN | 81 | 82 |
en_zh-TW | 54 | 55 |
Another method to understand the impact of source correction in the translation quality is through MQM-QE scores. In the table above, each LP has an average MQM-QE score from all segments from both datasets – ‘Original source’ and ‘Corrected source’. In all LPs, the MQM-QE scores from the ‘Corrected source’ were higher from 1 to 3 points.
To summarize, Unbabel has demonstrated a growing interest in the quality of both source and monolingual texts, leading to the development of our own typology known as Monolingual Typology. This typology is tailored to address both use cases – Localization and Customer Support – and enables us to automatically identify and categorize the errors and linguistic structures found in the text and also understand how those will affect the quality of the translation. Moreover, by incorporating automated source text correction, we can conduct a new quality management process that effectively enhances and ensures the quality of our final translations in a more streamlined and efficient manner.
See the power of Unbabel for yourself!
The work presented in this article was developed and written in collaboration with Marianna Buchicchio, Mariana Cabeça and Marjolene Paulo, Computational Linguists and part of the AI Product Team at Unbabel.